Deep representation learning and statistical physics in animal signal-receiver dynamics.
Doctoral defense by Ian Etheredge
- Date: Jul 5, 2021
- Time: 02:00 PM - 04:30 PM (Local Time Germany)
- Speaker: Ian Etheredge
- Location: Online oral examination
- Room: Online
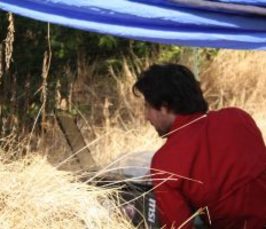
Signal-receiver dynamics underlie some of the most fundamental behavioral processes in individuals, groups, and ecosystems. They are also some of the most complex and difficult to understand. Fundamental to this understanding is to view signals through the eyes of their beholder, in reference to the specific perceptual experience of the study system. Throughout the history of sensory ecology and behavioral ecology research, we have attempted to improve our measurements in terms of the selective forces acting on them. In order to develop and test hypotheses, this has necessitated clever abstractions and distillations and much of the basis of ethology is in how we do this in principled ways (i.e., Tinbergen's four questions). More recently, advances in computational approaches (i.e., computer vision and deep neural networks) are changing the way we measure and manipulate data across fields and disciplines.
Principle among these is in providing higher resolution data as well as more expressive models capable of capturing more complex relationships. These techniques provide a novel opportunity to address the inherent complexity of many natural systems, signals, and behaviors. Still, how best to leverage these approaches and build scientific interpretation that informs existing ethological theory is not well established. Here we develop a novel framework for measuring animal signals using deep generative modelling which better captures complex relationships between signals and allows for developing more integrative studies. Then, using high-resolution descriptions of movement behavior, we borrow theory from statistical physics to understand the adaptive significance of traits using data driven, unsupervised measurements of behavior. And finally, we develop a new approach for measuring behavioral dynamics that scales to even large datasets, providing a robust, quantitative connection between movement signals and color-patterns. Together, the tools and techniques developed here provide a new framework which removes the necessity for human interventions and enables wholly new possibilities for testing and generating hypotheses related to signal-receiver dynamics and their evolution.